Kai Rothauge: AI Summit 2022
- Kai Rothauge
- Jan 12, 2023
- 2 min read
Kai Rothauge is CTO at Windjammer Technologies where he leads Spark Native Execution and cloud store checkpoint fault tolerance. Prior to Windjammer, Kai was a Postdoctoral Researcher at University of California, Berkeley in the RISELab where he developed Alchemist to accelerate Spark for Large-Scale Data Analysis by offloading to High-Performance Computing Libraries. Kai received his PhD from the University of British Columbia in Applied Mathematics, and his MMath from the University of Bath. Prior to his doctoral studies he also completed scientific internships at the Max Planck Institute, the Fraunhofer Institute, and CSIRO.
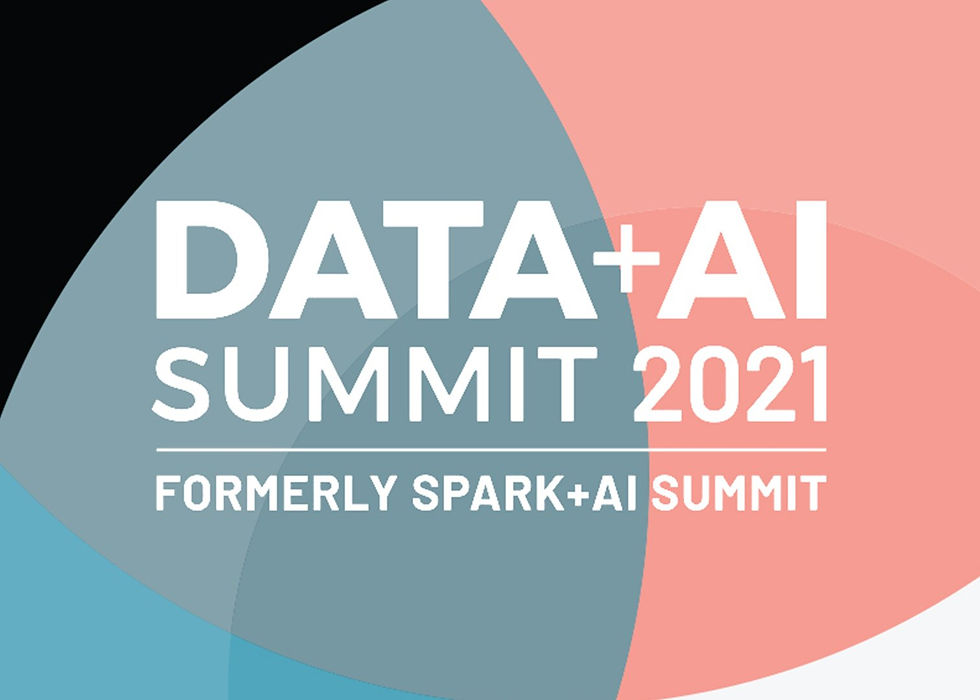
Why Spark Acceleration?

Spark doesn't fully exploit the high bandwidth of today's cloud storage system and is very CPU intensive. The result is an increased number of required worker nodes, performance instability, management challenges, and high query run times. The Apache Spark fault tolerance requires persisting data at shuffle boundaries, and motivates complex and expensive shuffle services.
Our Engine:
SNE optimizes Apache Spark on disaggregated compute clusters with cloud store based Data Lakes.
- Efficient use of expensive CPU resources: Native execution, MPP (massively parallel processing) dataflow clustered architecture eliminating JVM and Map-Reduce bottlenecks
- Exploits cloud storage bandwidth: Aggressive, parallel precise prefetch to retrieve data from cloud store Data Lakes
- Eliminates need for complex shuffle service: Spill and checkpoint-based fault-tolerance uses reliable, high bandwidth cloud storage: no need for special shuffle service while providing full query fault tolerance including spot instance and cluster interruptions.
- Transparent, 100% compatible
Windjammer is a solution that is 100% Apache Spark compatible that transparently addresses the performance, cost, and predictability limitations when running Spark with Data Lakes in cloud stores.
SNE Spark Integration
- Windjammer Transparently plugs into Spark as a SparkSQL extension
- Plugs in as a thin wrapper to plan optimizers through spark.sql.extensions
- Transparent to all query submission interfaces such as Spark-submit, Spark shell, Spark-SQL shell, notebooks, etc.
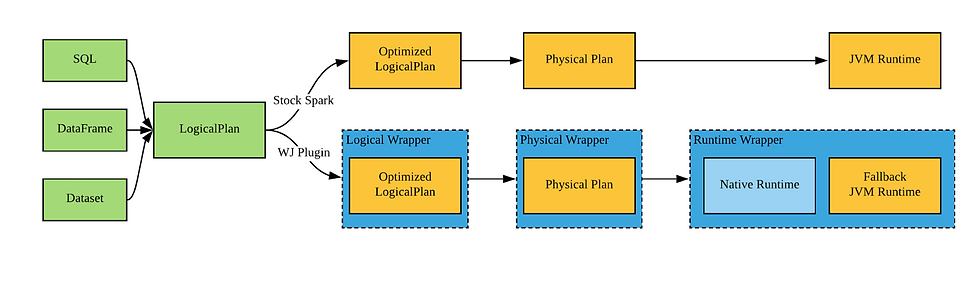
- Wrapper directs the query to WJ spark native execution engine (SNE)
- Queries transparently fall back to stock Spark JVM execution runtime engine if native execution unable to accelerate
-All queries complete with exact semantics of stock Spark
- Deployed as a JAR file with single-click installation
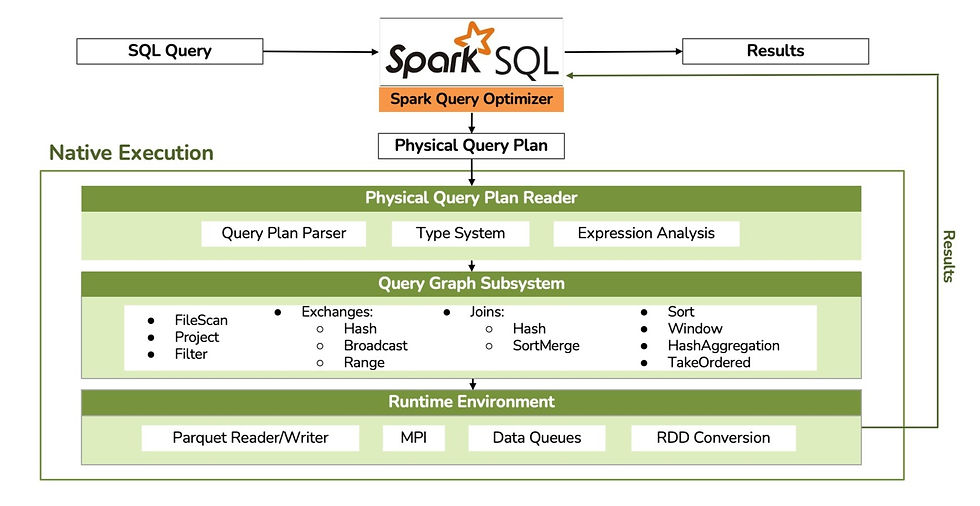
Comments